Course Description
Genomic, transcriptomic, proteomic and metabolomic, collectively “omics” data, are being generated on large cohorts of individuals at an unprecedented rate. These data are transformed through statistical means into risk prediction models, giving insights into health and disease.
This course was developed to give life science and related professionals a baseline understanding of and the ability to critically evaluate biomedical risk prediction models for use in medicine.
The course is designed for non-statisticians, but assumes students will have some familiarity with interpreting statistical results. The material draws on diverse fields (Biostatistics, Machine Learning, High-dimensional statistics, Epidemiology, Decision Theory), making it unique and highly relevant to the development of precision medicine.
The online format means you can learn at your own pace, but we also offer direct access to the course instructor via live webinar office hours every other week.
Have you ever found yourself in any of these situations?
- You’re reading a paper about a new risk predictor based on omics data and while the results are compelling, you really have no idea how to evaluate whether the statistical methods are valid.
- You run a large research group and need to present the results of some omics data analysis to upper management. The only problem is that you’re not fluent in statistics, making it difficult to get the most out of conversations with your analysts and to be able to distill the findings down for management.
- You see an advertisement for a new molecular diagnostic test that claims to be able to predict disease recurrance. You want to evaluate the evidence behind the test, but you have no idea how to make sense of the data presented.
Prediction Models in Omics was designed to educate life science professionals about the different approaches used to develop omics-based prediction models. This course is for:
- Researchers in academia, research institutes, pharma, biotech or molecular diagnostics companies, who are using omics data to develop predictive tests for clinical use
- Physician-scientists working in academic medicine
- Biological scientists who generate different types of omics data used in prediction models
- Computational scientists seeking a high-level overview of prediction models
- Healthcare providers who want to be able to critically evaluate commercially available omics-based molecular diagnostic tests
- Software companies developing tools to implement risk calculators
- Regulators or insurance companies who want to evaluate omics-based risk prediction products
- Students who can’t find an equivalent cross-disciplinary, practical course available at their academic institution.
What you’ll learn in the course
Prediction versus association/causal effects
Common uses of statistical modeling in biomedicine are to characterize associations between molecular data and clinical features of disease and also to make predictions (risk estimation or classification). While these two goals differ in many important aspects, the language used to describe results for each is often used interchangeably. Understanding the differences in these two goals allows one to properly evaluate results from omics-based research. This lesson lays the foundation for the rest of the course.
Performance metrics to evaluate prediction models
After establishing how models for association/etiology differ from prediction, we move onto what performance metrics characterize prediction and the motivation for using these metrics. For example, we discuss why established metrics for association, such as odds ratios, are not suitable for making predictions.
Estimating unbiased performance metrics (Resampling)
When estimating predictive accuracy, bias can distort the results, leading to an overstatement of the model’s accuracy. There are many ways for bias to arise, especially in high-dimensional settings in which thousands of gene-expression measurements may be made. We will discuss how techniques such as cross-validation allow unbiased estimation. A series of simulation studies will be used to demonstrate concepts.
Design and generalizability
After covering how to evaluate predictions, we move onto design-related aspects including the impact of sample size, sampling, and generalizability. We use examples from the literature to bring intuition to the potentially abstract concept of generalizability.
More performance metrics
In addition to accuracy, an equally important measure of performance is calibration. This is discussed less frequently, but it is equally important. Furthermore, an evolving area of research is developing metrics that incorporate the consequences of a decision. That is, how to incorporate the value of true positives versus false positives. This can be to further characterize the usefulness of a diagnostic tool as well as motivating the need for a trial to assess the benefits of a prediction model.
Aspects unique to Omics & replication problems
There are aspects of developing prediction models that are unique to omics. For example, the initial discovery data is usually not the platform for final assay. Challenges with replication must be acknowledged. We will see how most of the documented reasons why prediction models fail relate to points made during this course.
What our students are saying
“Excellent course for introducing relevant topics to my work and opportunity to take deeper dive into specific issues on these topics. Dr. Suchindran is very knowledgeable and excellent teacher; his presentation was clear and engaging”
“Sunil’s lecture is very impressive and helpful. It helps me understand how to use the appropriate model for risk prediction and classification in the omics setting”
“Great job handling questions and making material interesting.”
“The presenter did a great job introducing key concepts and vocabulary and the purpose and place for each one in research applications. There isn’t a current application in my research, but will definitely pursue more knowledge in some of these topics for future applications of interest. Thank you for your time and preparation!”
“I now understand these concepts much better and have the right words to think through biomarker studies and concepts.”
About the course
Prediction Models in Omics consists of the following:
- 8 on-demand video lessons (total watch time ~4.5 hours)
- Live, interactive office hours via webinar every other week
- An active online discussion forum
- Reading list including key papers
Please note: We recommend that students taking the Prediction Models in Omics course have a basic understanding of statistics before enrolling. The course is designed for non-statisticians, but assumes attendees have some familiarity with interpreting statistical results.
Course Price
This course is available for free
All materials are copyrighted. Republication or redistribution of PMA content is prohibited without prior written consent. PMA shall not be liable for any errors or delays in the content, or for any actions taken in reliance thereon.
Instructor
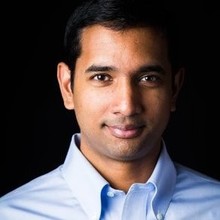
Senior Biostatistician, Duke University Sunil Suchindran, PhD
Course curriculum
-
-
Pre-course survey
-
-
-
Introduction to course (9 min)
-
How to evaluate risk prediction methods (20 min)
-
Predictive versus association/causal effects (39 min)
-
Performance metrics to evaluate prediction models (28 min)
-
Estimating unbiased performance metrics (Resampling) (51 min)
-
Performance metrics - calibration (28 min)
-
Design and generalizability (19 min)
-
Performance metrics - decision curves (26 min)
-
Aspects unique to Omics & replication problems (32 min)
-
-
-
Reference list
-
-
-
Tell us about yourself
-

About this course
- Free
- 12 lessons
- 4 hours of video content
Reviews
Excellent succinct course even for non-statisticians
Vanessa Gonzalez-Covarrubias
The organizacion, peace and content was very good, I learned the basics to perform rational study designs and model development through its various steps tow...
Read MoreThe organizacion, peace and content was very good, I learned the basics to perform rational study designs and model development through its various steps towards their usefulness and validity. Highly recommended.
Read Less